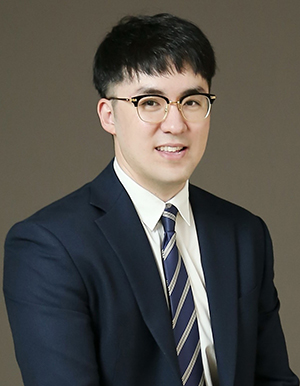
Note: Coffee and snacks will precede the seminar at 3 p.m. in the Ford ES&T First-floor atrium
Joseph Kwon, Associate Professor, Texas A&M University
"Transformer-based Hybrid Modeling and Control of Evolving, Nonlinear Processes"
Abstract:
Traditionally, the dynamic modeling of chemical processes has relied on first-principles models grounded in fundamental physics and chemistry laws. These models, primarily formulated through differential equations with constant parameters, enable the calculation of control actions optimizing process operations, taking both process and actuator limitations into account. However, the ever-evolving and nonlinear nature of chemical processes frequently calls for models with time-varying parameters. Relying on constant-parameter models for control of complex process systems, especially those with evolving behaviors, poses significant challenges. For example, factors such as the presence of microorganisms in large-scale fermentation or unaccounted factors causing performance degradation can limit the applicability of first-principles modeling with constant parameters. In response, data-driven models, notably recurrent neural networks, offer a means to capture such evolving dynamics. However, they come with their own set of issues, including interpretability, extrapolation limitations, data dependency, and the risk of overfitting, which have limited their widespread adoption.
Motivated by these challenges, we have developed hybrid models that integrate system-agnostic first-principles dynamics with system-specific data-driven, time-varying parameters. This approach offers a harmonious 'best of both worlds' modeling solution for chemical processes whose dynamics change due to some uncertain reasons. Specifically, current system states, such as concentration and temperature, are harnessed to estimate kinetic parameters like rate constants. These parameters are then integrated with the dynamic process model rooted in first-principles. Our hybrid modeling framework incorporates a recent innovation: attention-based time-series transformers (TSTs) coupled with positional encoding. This marks a pioneering venture into applying the transformer algorithm – a cornerstone in ChatGPT’s triumph - to nonlinear, time-varying processes. By analyzing data across both current and preceding time steps, the TST captures both immediate and historical changes in process states, granting a contextual insight on process dynamics, mirroring ChatGPT’s textual context understanding. This TST-based hybrid model identifies correlations between process parameters and state variables. Its versatility is evident as it adapts to a spectrum of models - from density function theory to computational fluid dynamics - and scales, spanning from laboratory to extensive industrial environments. We have also leveraged these TST-based hybrid models to design soft-sensors for estimating unobserved process states and to formulate model-based controllers. We will present applications of this hybrid modeling and control architecture, showcasing its utility from labs to industrial processes, made possible through partnerships with leading chemical process enterprises.
Bio:
Joseph S. Kwon is an Associate Professor in the Department of Chemical Engineering at Texas A&M University (TAMU), where he holds the esteemed Kenneth R. Hall Career Development Professorship. He earned his B.S. in Mathematics and Chemical Engineering from the University of Minnesota, Twin Cities. Subsequently, he obtained his M.S. in Electrical Engineering from the University of Pennsylvania and a Ph.D. in Chemical Engineering from University of California, Los Angeles. Dr. Kwon specializes in the development of computational models and frameworks within the field of Process Systems Engineering (PSE). His research focus encompasses multiscale modeling, hybrid modeling, model predictive control, and optimization for diverse chemical and biological process systems. He has published over 120 papers in top-tier journals, and delivered numerous invited talks at prominent chemical process entities such as ExxonMobil and Halliburton, universities, and conferences. To date, he has secured over $4 million from agencies like the NSF, DOE, as well as from private industry. He has received multiple awards, including the Hanwha Non-tenured Faculty Award, KSEA Young Investigator Award, KIChE President Young Investigator Award, and James M. Lee Memorial Award. Within TAMU, he has received the Engineering Genesis Award, TEES Young Faculty Fellow Award, and Distinguished Teaching Award from the Department of Chemical Engineering. Additionally, he has secured best paper awards from both the IET Systems Biology Journal and the Mathematics Journal.